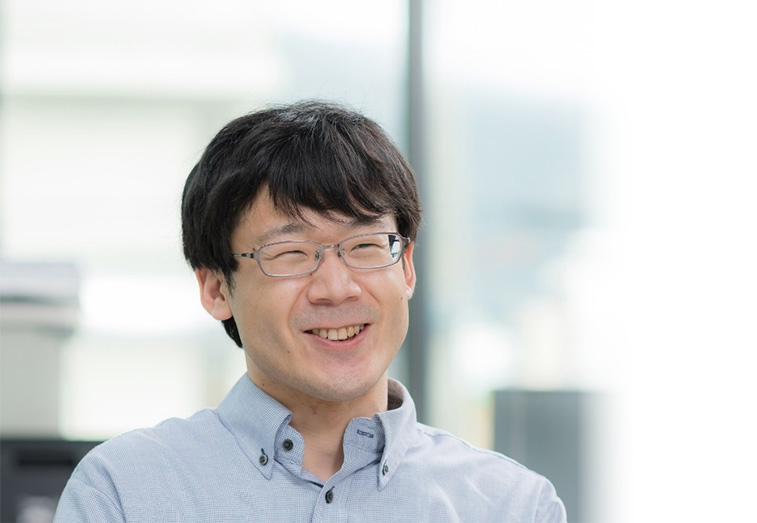
マルチモーダルAI基盤技術研究チームでは、画像、自然言語、音響信号、時系列データ、構造データなど、さまざまなモダリティや階層データを統合的に扱うAI技術の開発を行い、それらを応用して生命科学における多様な課題の解決に取り組んでいます。具体的には、複雑なデータを大規模に扱うためのモデリング技術やその基盤技術の開発に注力し、それらの成果をツールやプラットフォームの開発に結びつけ、実際のアプリケーションへの展開を目指しています。
研究テーマ
- 各モダリティの大規模モデルの技術開発および手法・理論研究
- 大規模マルチモーダルモデルのための技術開発および手法・理論研究
- 現場での大規模モデル活用に向けたツール・プラットフォームの研究開発
主要論文
Kojima R, Okamoto Y.
Learning deep input-output stable dynamics.
Advances in Neural Information Processing Systems
35, 8187-8198 (2022)
doi: 10.48550/arXiv.2206.13093
Ishida S, Terayama K, Kojima R, et al.
AI-Driven Synthetic Route Design Incorporated with Retrosynthesis Knowledge.
Journal of Chemical Information and Modeling
62(6), 1357-1367 (2022)
doi: 10.1021/acs.jcim.1c01074
Nakamura K, Kojima R, Uchino E, et al.
Health improvement framework for actionable treatment planning using a surrogate Bayesian model.
Nature Communications
12(1), 3088 (2021)
doi: 10.1038/s41467-021-23319-1
Kojima R, Ishida S, Ohta M, et al.
kGCN: a graph-based deep learning framework for chemical structures.
Journal of Cheminformatics
12(1), 32 (2020)
doi: 10.1186/s13321-020-00435-6
Kojima R, Sugiyama O, Hoshiba K, et al.
HARK-Bird-Box: A Portable Real-Time Bird Song Scene Analysis System
2018 IEEE/RSJ International Conference on Intelligent Robots and Systems
(2018)
doi: 10.1109/IROS.2018.8594070
Kojima R, Sato T.
Learning to rank in PRISM
International Journal of Approximate Reasoning
93, 561-577 (2018)
doi: 10.1016/j.ijar.2017.11.011
Kojima R, Sugiyama O, Suzuki R, et al.
Semi-Automatic Bird Song Analysis by Spatial-Cue-Based Integration of Sound Source Detection, Localization, Separation, and Identification.
2016 IEEE/RSJ International Conference on Intelligent Robots and Systems
(2016)
doi: 10.1109/IROS.2016.7759213
Kojima R, Sato T.
Goal and Plan Recognition via Parse Trees Using Prefix and Infix Probability Computation
In: Davis J, Ramon J (eds) Inductive Logic Programming, Springer
(2015)
doi: 10.1007/978-3-319-23708-4_6
メンバー
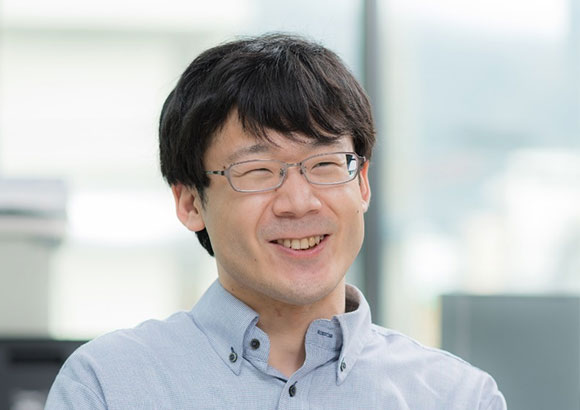
チームディレクター小島 諒介
- ryosuke.kojima@riken.jp
- google scholar